Why can’t we find the 50% of people with rare diseases?
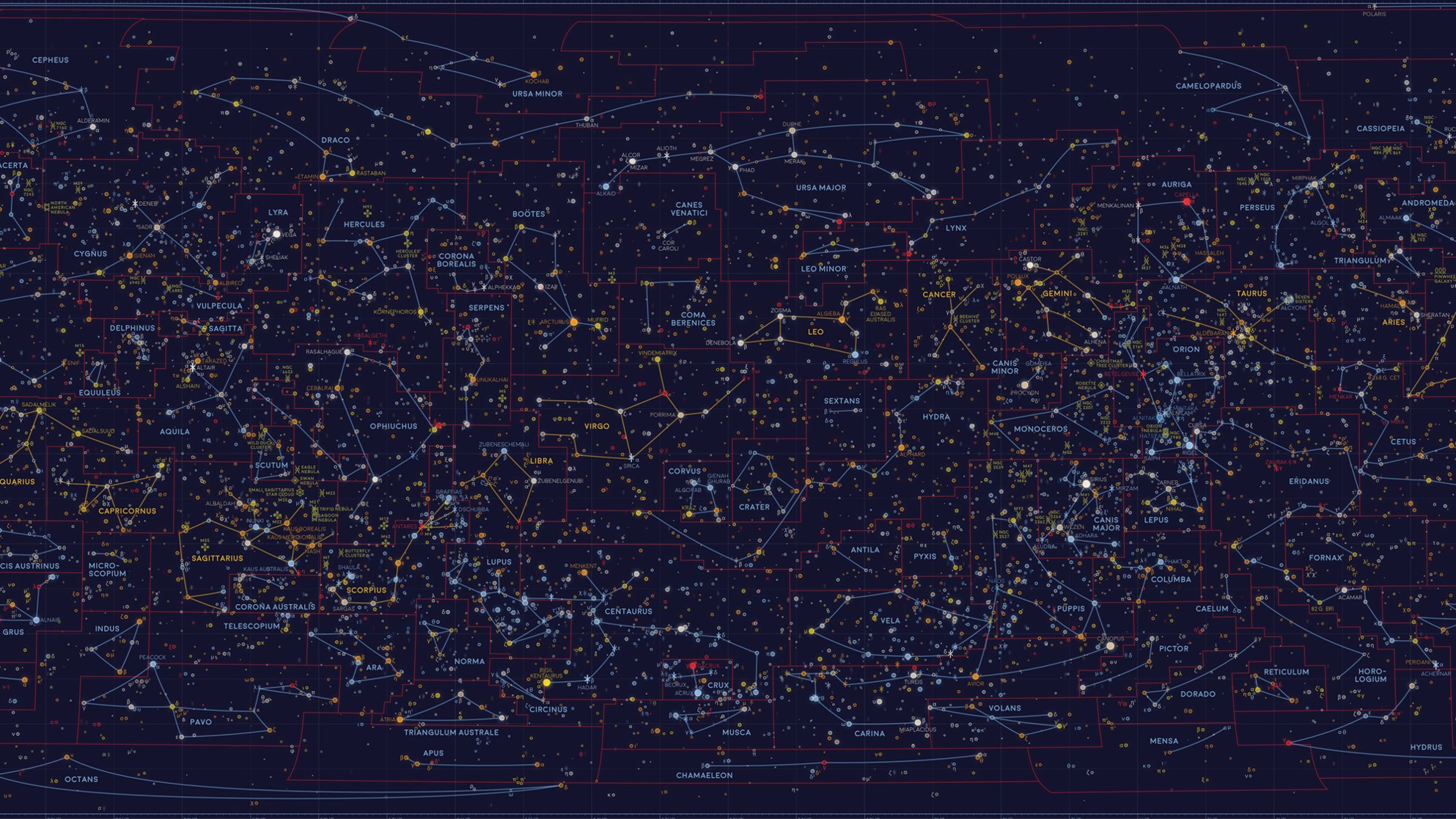
It might be said that picking out patterns to identify patients with rare diseases is a bit like distinguishing thousands of constellations of stars. Neither is within the scope of the human eye and both require extremely advanced technologies to even begin to decipher and separate patterns. Yet finding the 50 percent of undiagnosed patients with one of the approximately 7,000 rare diseases is a medical and clinical imperative.
Typically, the way clinicians diagnose patients is by taking what the broader healthcare industry knows about a disease – generally as described by key opinion leaders (KOL) – and correlating a patient’s symptoms to those definitions. The problem with this approach when it comes to rare and ultra-rare diseases is that it is subject to experiential bias. If the KOL has not observed a pattern of symptoms or the order in which those symptoms emerge differs significantly, the patient will likely remain undiagnosed.
There is so much we don’t know about rare disease, but what we do know is that there is enormous heterogeneity of symptoms – so much so that as many as 60% of rare diseases present with significant heterogeneity, according to genomics experts. Understanding this 60% variation in symptoms with rare diseases is undoubtedly the greatest challenge facing both healthcare professionals as well as the companies seeking to find and develop new treatment options. Even for those rare diseases where there are already treatments, the difficulty can be diagnosing patients early enough to limit the worst effects of the disease. For example, some symptoms may not be flagged as significant from a clinical perspective, despite the challenges they present to the patient on their journey to diagnosis, and by the time the patient’s symptoms escalate to correlate with recognised patterns, it’s often much later in the disease’s progression, on average, six years from the onset of symptoms.
Connecting local and global
Let’s consider the types of data that contribute to an understanding of a patient’s symptoms and possible diagnosis.
The electronic medical record contains important data that contributes to a broader understanding of the patient and their journey, including diagnostic codes, medication codes, clinical measurements, and clinical notes to ascertain whether a person has the target disease
Beyond patient-specific information, it’s important to also consider factors that contribute to data about a rare disease, including genetic disease versus de novo mutations versus environmental factors. During a panel discussion at the World Orphan Drug Congress 2020, Ron Herings, Ph.D., director and founder of independent research organisation PHARMO Institute noted that data gathered at a local level can help to pinpoint prevalence of genetic disease, often tracing those with the disease to small villages and to people with a common ancestor. This can help to provide valuable insights into rare diseases and even trace potential patients, based on ancestry.
Some rare and ultra-rare diseases, however, are de novo mutations of a disease with no apparent genetic correlations, as Raymond Huml, Vice President Medical and Scientific Strategy, Head of the Rare Disease Consortium at Syneos Health Clinical Solutions pointed out during the panel discussion. In these cases, AI models and large databases and registries are important for finding such patients.
Medication response and medication adherence provide other important insights that can help to flag and pinpoint phenotypic differences in a rare disease. For example, if a patient has been diagnosed with multiple sclerosis based on symptoms but does not respond to the preferred treatment, he or she may well have a sub-category of the disease and could be better suited to a different therapy.
The insights that the AI model gathers about these patients can help to expand knowledge about phenotypic differences and allow clinicians to better diagnose and treat patients, while also enabling pharmaceutical companies to better identify potential patients for new therapies.
The Volv Approach to finding the 50%
Despite growing knowledge about rare diseases, the problem of finding the undiagnosed 50 percent of patients remains. Most machine learning models fail to achieve this objective because of the limited number of already diagnosed, ‘labelled’ patients from which to learn about the disease.
To address this challenge, Volv has taken a very different approach by using a novel algorithm that adds unlabelled or unreliably labelled patient data to the learning procedure.
What we have found through our unique, proprietary AI model for finding undiagnosed and misdiagnosed patients, InTrigue, is that diagnostic codes for rare diseases are very often wrong for rare diseases. We also found significant gaps in the data, which needed to be addressed. Unlike other approaches, Volv’s model learns more about the disease through analysis of certain key data points with the EMR, including symptom prioritisation, the patient journey, misdiagnosis and variance in clinical decision making. It also gathers learnings from patient registries, clinical trials and other sources.
If we return to the premise of identifying those many different constellations – or the proverbial finding a needle in a haystack – and compare that to finding patients with rare diseases, we vastly improve the odds by scanning data points to around 9,000 or 10,000 individual measurable properties in the dataset, overlaid with 75 features from the EMR – medication code, clinical measurements, clinical notes, etc., to uncover underlying biomarkers and predictors.
By way of example, we ran our methodology inTrigue, across acute hepatic porphyria (AHP) to find undiagnosed patients. We compared our methods with other standard machine learning methodologies that have been published with real-world evidence teams in PubMed and found significant differences. Other methods would need a cohort of 50,000 to be confident of a single AHP patient. Our methodology inTrigue finds one patient for every 1.27 flagged by our algorithm.
Why the 50% matter
Finding undiagnosed patients matters for so many reasons. Most importantly, it matters to the patients and their carers who are struggling to get a diagnosis and – wherever possible – a treatment or cure. It matters to the clinicians who spend countless hours and resources trying to help patients who present with complex and poorly understood symptoms. It matters to patient advocacy groups who need the support and input that will help them better support patients, lobby for funding and work with drug developers to share their knowledge on symptoms and patient end points. It matters to healthcare systems that are struggling with escalating costs since good data means fewer unnecessary genetic tests. And it matters to the pharmaceutical companies and biotech companies that are developing products to treat and cure rare diseases, who need to recruit patients for clinical trials and who need to know their markets and demonstrate the medical and economic value of their products.