The Path to Rare Disease Clinical Trial Innovation
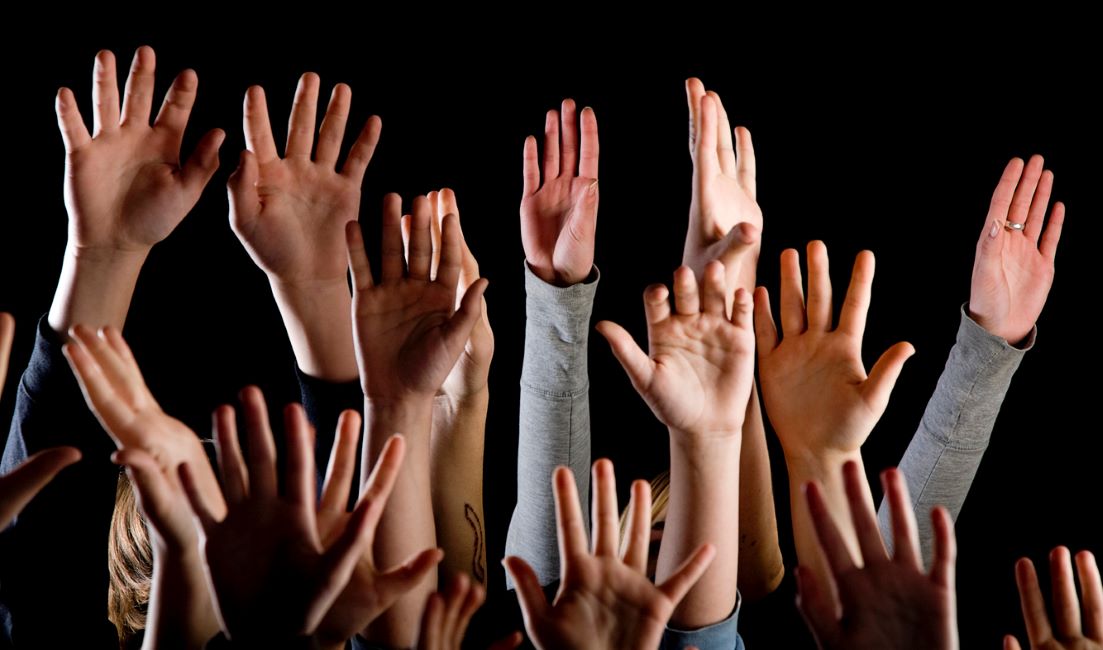
Credit Photo by RichLegg on iStock
Executive Summary
For decades, the pharmaceutical industry has faced the same recurring problems with clinical development: the struggle to fully recruit and retain enough patients, meet target timelines, and have trials conclude on time.
Certainly, the industry does overestimate its ability to recruit, but a bigger issue is that study designs and protocol development seemingly fail to truly reflect patients’ lives, or account for the reality in the clinic. In fact, data shows the probability of success for any clinical development effort is 6.2% for orphan drug trials, compared with 13.8% overall, which translates to a 93.8% failure rate for orphan drug development efforts.
Given the often progressive and irreversible nature of rare diseases, there is a need to increase efforts to find those undiagnosed patients, diagnose them earlier, and bring them into the frame when developing new treatment options. To achieve this, collectively as an industry, we must do more research into the rare disease patient population to characterise and better understand both the already diagnosed and the undiagnosed. We need this deeper understanding before deciding on the best clinical development strategy, finalising clinical trial design, and starting the enrolment of the patient population in a clinical study.
To do that, clinical researchers and drug developers need to include much more knowledge and understanding of those people who are unknowingly living with the disease in the design of clinical development plans and study protocols. To find those people, there is a need to consult more extensively on the design of protocols, not just with the key opinion leaders, but also with physicians that are typically seeing and treating larger numbers of patients.
One crucial factor with rare diseases is that the diagnostic journey is arduous and lengthy, often with many patients not being correctly diagnosed. As an example, a study found that 58% of Ehlers-Danlos syndrome (EDS) patients consulted more than five doctors, and 20% consulted more than 20[i].
So, when designing and recruiting for clinical trials, drug developers must first learn where the "as yet undiagnosed patients" are "hidden" – in other words, where they may be in the healthcare system, and which specialists they are seeing. It is those specialisms that need to be brought along in the diagnostic journey, so they can learn to identify rare disease patients within their practice. This is very well illustrated in the case of acute hepatic porphyria (AHP), where the view is that patients reside in the gastroenterology world, but, in fact, an even larger group is residing in other specialties. Another example is cited in Chapter 2.
With novel approaches, such as the use of Machine Learning (ML), we can now highlight people who are not yet diagnosed as patients but are likely to be living with a disease, for their clinicians’ attention. Subtle indicators are derived from health care records by using ML, which would be difficult or nigh impossible for a doctor to recognise amidst the wealth of data already in front of them.
Conducting thorough natural history studies of patients living with disease, but also including those wider populations of people suspected of living with disease but currently undiagnosed, can help to uncover sentinel events or detectable physiologic changes that are key predictors of disease progression or that are clinically important. These can provide an understanding of which subgroups of people living with the disease might benefit from a drug in development and should therefore be targeted for inclusion in the clinical trial.
And, importantly, clinical researchers need to scrutinise the data and adopt insights gained by using ML models which will enable better clinical development strategy, design, and patient stratification.
First, though, we need to understand the barriers and misconceptions about the art of the possible and address those directly.
This paper explores the changing expectations of the regulators, the challenges the health industry continues to face, and the ways in which we can rethink the entire clinical development process – from development strategy to protocol design, to patient identification and recruitment – to achieve real breakthroughs in rare disease research and development.
Chapter 2: Misconceptions and industry challenges
The path to rare disease innovation begins with a better understanding of the complexity of each disease – a point well understood by the health authorities.
As the US Federal Food and Drug Administration (FDA) has identified in its guidance on natural history studies, rare diseases can have substantial genotypic and/or phenotypic heterogeneity. As such, the natural history of each subtype, if it exists at all, may be poorly understood or inadequately characterised. Above all, a typical natural history study certainly does not include those people living with the disease that – in rare - often remain undiagnosed.
There are two levels of undiagnosed patients: those who have had no diagnosis at all and have therefore not been matched with a disease, and those who have had a partial diagnosis but whose symptoms are not well characterised and therefore do not belong in a defined subgroup. As researchers learn more about rare diseases, they are starting to understand that different phenotypes may present with the involvement of different organ systems, with varying degrees of severity or rate of deterioration.
As noted earlier, ML can help to elicit subtle indicators from electronic health records or claims data. However, during panel debates at recent orphan drug conferences, there seemed a strong bias towards the use of registries for research and patient characterisation, and there were clear misconceptions from both industry and regulators about the usability of primary care electronic medical records (or electronic claims data) for the purpose of early disease detection, be it in a traditional manner, or ML assisted.
The limitations of registries
While disease registries have a clear purpose, they are constrained by the fact that they tend only to contain data on patients that are known to have a given disease. By focusing only on rare disease data that already exists in patient registries, research and potential patient populations remain excluded from further studies.
There are other limitations to registries too. Funding for disease-specific registries is often lacking and the registry data can be unreliable. Often there is poor interoperability between different registries within the same disease state, although there are exceptions.
These challenges could be mitigated with more stakeholder engagement and collaboration, as well as a commitment to disease-specific rather than product-specific registries. This might include coordination between pharmaceutical companies, hospitals, and patient organisations to establish and manage disease registries. As an example, the Loulou Foundation worked with seven biopharmaceutical companies to coordinate an observational study on CDKL5 deficiency disorder[ii].
The challenge with patient heterogeneity
Another issue occurs during clinical trial patient recruitment, particularly given the high degree of heterogeneity within some rare diseases.
If trial recruitment is based largely on what is known in patient registries, the study will miss out on the wealth of information about the disease from undiagnosed patients. If, on the other hand, recruitment starts from a more general population with pre-defined inclusion and exclusion criteria based on what is known about the disease, it is likely the study will end up recruiting patients with previously undiscovered heterogeneous characteristics. If not carefully planned, this will only become apparent when data shows patients responding in mixed ways. The objective, while difficult to achieve, should be to discover more about potential heterogeneity in the patients to incorporate that knowledge into the clinical development and trial designs to avoid a failed study with some underpowered but promising subgroup analysis.
It needs to be recognised that getting a diagnosis can take months, years, or, in some cases, a patient’s entire life. As has been noted, “around 50 percent of patients with a rare disease remain undiagnosed even in advanced expert clinical settings where Whole Exome Sequencing (WES) is applied routinely as a diagnostic approach[iii].”
Volv’s own work confirms this. For example, acute hepatic porphyria (AHP), a group of rare genetic conditions that can cause severe, acute symptoms, is reported to have a prevalence of 5 to 10 cases per 100,000. These patients are largely diagnosed by gastroenterologists. However, Volv’s real-world evidence research on EMR data indicates that 52.5 % of patients are missing their diagnosis, which may in part be explained by the fact their primary lived patient experience doesn’t match at all with the prevailing understanding of them being seen in gastroenterology but revolves primarily around being seen in psychotherapy, neurology, obstetrics, and gynaecology.
Making sense of heterogeneous patient data
One further challenge is that patient data tends to exist in multiple different systems. The ideal would be to have data consortium for rare diseases. There are, however, operational barriers to creating such consortia because different healthcare institutions and hospitals have their own EMR systems, their own governance programmes, and differences in how data is coded into medical dictionaries.
There are also differences between how healthcare systems carry out training and education, their reimbursement processes, and even some guidelines, e.g., how they test for a rare disease.
However, these differences can be accounted for when the right processes and solutions are in place. With the right tools and know-how, it is not only possible but desirable to search primary care electronic medical record data or claims data to find undiagnosed patients. In most healthcare systems, the most holistic and longitudinally rich set of data on any one individual resides in primary care systems. It is the general practitioner who refers patients to secondary and tertiary care, and, in a well-run system, there is a feedback loop from those specialists back to the GP.
So, to understand the patient and the patient's journey as a whole and discover what may be predictive of them having a disease – particularly with rare diseases with heterogeneous symptoms - researchers need to analyse all or part of the real-world patient data that is available to uncover people living with disease that are not yet correctly diagnosed. ML applied to primary care EMR, or electronic claims data can help with this otherwise humanly impossible task.
Chapter 3: Making better use of real-world data
The difficulties in finding rare disease patients are well-understood, but for that to change, there needs to be a shift in how people living with a disease are identified and included in the research effort towards developing new treatment options. Registries have their place, but they cannot be the only source of information.
While EMR data might be messy and incomplete, it is replete with insights on individuals that cannot be found in patient registries. Trying to curate that data into a registry and make it uniform means the data points that do not fit a prescribed pattern will be lost, and, as a result, patients with heterogenous symptoms will not be identified.
This is a common issue for people with rare diseases. Surveys have found that it can take as long as 7.5 years to obtain a rare disease diagnosis. Insufficient knowledge and data increase the risk of misdiagnosis for rare disease. For example, 56% of Ehlers-Danlos patients are misdiagnosed at some point in their diagnostic journey. Some rare disease patients receive up to 8 wrong diagnoses before reaching the right one. This is true in many rare diseases, causing significant burden for people living with rare disease, as various studies have shown[iv].
How, though, can you make sense of that messy, non-curated data?
A patient's medical record can contain more than 50,000 possible data points from which a clinician must discern the pattern of a disease. Furthermore, different phenotypes may present, with the involvement of different organ systems, with different severity or rate of deterioration.
The difficulty is compounded by several other factors. International Classification 11 (ICD-11) includes around 5,500 rare diseases and their synonyms. The list of rare diseases is regularly updated in close collaboration with Orphanet and with the WHO Collaborative Global Network for Rare Diseases (CGN4RD)[v].
Rare diseases are distributed across different chapters following a primarily clinical approach. The main code is selected according to the most severe system involvement or the specialist most likely to be relied on to manage the disease. The issue for individuals is that more than 9,000 rare diseases have been discovered, which means a substantial number do not even have a code to capture them, much less a treatment or cure.
Given this huge number of diseases, synonyms, and symptoms, identifying people with a rare disease, be it as a clinician or a researcher, is fraught. In the past, Volv has described this challenge as like trying to find one out of the thousands of constellations of stars in the night sky. Clinicians today are looking at the equivalent of more than 10 night skies to pick out individual constellations that represent the rare disease – most of which are unknown to them.
Deciphering this data manually is next to impossible. If, collectively, as HCPs, researchers, and drug developers, we want to address the problem of poorly identified patients – an issue that impacts the success of clinical trials and the ability to treat patients faster and more effectively – we must consider Machine Learning (ML) approaches.
Although it comes with its own challenges that need to be carefully managed, ML is particularly good at digesting large amounts of data very quickly and identifying patterns or finding anomalies or outliers in that data. As a result, an important application in healthcare is the development and implementation of more accurate clinical prediction models (algorithms, tools, or rules) to help clinicians to improve screening, diagnosis, and prediction of diseases.
Using ML to identify patients and inform the clinical development strategy ensures all data – meaning anyone who potentially might be living with a disease – is considered and analysed.
As an example, Volv conducted a retrospective study on failed clinical trial studies to better understand why they failed and assess potential candidates and biomarkers to predict responders and non-responders for each candidate. The study found that the Multiple Sclerosis (MS) population has subgroups that respond differently to treatment options and failure could be attributed to many different factors, including poor cohort selection and poor endpoint selection. Analysis of the studies produced 22 ‘core’ models with which to predict patient response to 15 MS therapies and facilitate personalised treatment optimisation[vi].
This is a well-known issue for many rare diseases. One problem is that sponsors must balance the added cost of more detailed characterisation against the risk of the trial failing. Since regulators do not insist on stratification, the decision to do so is left to the sponsor.
Educating the regulators
The FDA has taken a more progressive approach to natural history data and real-world data to advance knowledge of rare diseases, identify patient populations and establish clinical outcome assessments and biomarkers.
Both the FDA and the European Medicines Agency (EMA) also recognise the importance of real-world data (RWD) and real-world evidence (RWE) to inform decision-making. Under its 21st Century Cures Act (Public Law 114-225), the FDA established an RWE programme that looks at how to use it in regulatory decision-making.
To use RWD effectively and appropriately in clinical research and development - be it for recruitment, outcome assessments, or overall RWE generation - the application of ML is playing an increasingly vital role.
As the data science field advances and its adoption becomes more widespread, and as new standards emerge, there is a need to educate and collaborate with the regulators on the art of what is possible so that the regulations and guidelines remain relevant.
A joint Big Data Task Force from Heads of Medicine and the EMA seeks to support regulators and industry in realising the potential of big data in public health and innovation. As a report from the task force notes, “it is clear that the data landscape is evolving, and that the regulatory system also needs to evolve, and to prepare for and understand the diversification in data generation and knowledge management that will be required[vii]."
Rethinking endpoints
Beyond the issue of finding the right patients for a rare disease clinical trial, there is a need to identify or develop clinical outcome assessments, considering what is relevant for the patient population.
A key objective should be to map out the expression of a disease early in its progression by uncovering poorly recognised characteristics of the disease. Those characteristics may serve as novel clinical trial endpoints that may have more relevance to the patient population than already established ones.
As an example, in neuro-muscular studies, the 6-minute walk test is widely used and is validated, but is not considered to be a good assessment of how the patient is doing.
Those endpoints need to be confirmed and validated with patients living with the disease, so sponsors must make sure they engage patients and caregivers in the design, assessment, and validation of those endpoints. This can be achieved using wearable devices to collect data on patient experience. Having endpoints that are relevant to patients, based on real-world evidence, will ensure early engagement, and encourage participation in clinical trials. It should also be noted that many of the patients with a rare disease are children or young persons whose illness also has an impact on their parents. This population of patients and their families have sometimes very different and distinct needs from adult patients, and they should be considered specifically for example, through Young Persons Advisory Groups (YPAGs).
For example, with Pompe disease, new measurable endpoints could include assessing whether the individual uses their hands to rise from a chair, whether they have difficulty reaching for objects above their head and washing their hair, as well as the use of codes to detect more generalised symptoms such as gait abnormalities and problems with mobility.
Importantly, when constructing new endpoints, there will need to be early involvement and buy-in from the regulators to ensure the proposed endpoint does indeed measure what it is designed to, and that it is considered an acceptable parameter by the health authorities.
If patient data can uncover a person living with a disease earlier, it may be possible to focus on the expression of that disease in its earlier stages - which may be different from symptoms later in disease progression - and then try to address those issues. The earlier recognition of a rare disease might also help to mitigate the disease progression.
Paving the way to better outcomes
There is much that needs to change if the challenges with rare disease clinical trials are to be overcome. By making use of patient data to understand disease patterns and development earlier and exploring new endpoints of relevance to patients, it will be possible to ascertain which patient populations are more likely to respond to a particular treatment.
Chapter 4: Where to from here?
The problems with identifying and recruiting patients with rare diseases as well as advancing innovative products through clinical trials can be addressed with better use and understanding of all the data relevant to both diagnosed and undiagnosed patients.
This can be achieved through an ML-empowered approach that uses extensive, anonymous patient data to select a more precise target patient population for clinical development.
The goal should be to gain a deeper understanding of the disease in question, including heterogeneity of symptoms, and the differences in patient-lived clinical experiences. Any ML models and protocols should embed patient insights and expertise in a planned way to truly revolutionise the way trials are carried out.
With this in mind, we can then develop more specific and relevant endpoints for the target indication and product profile, and to better understand the mechanism of action through the development of new biomarkers. ML can be of significant help in achieving these goals.
There are no simple solutions to improving outcomes with clinical trials for rare diseases; however, there are tools that can take innovators a step closer to achieving the goal of finding and developing treatments and cures that will work for patients living with rare diseases.
Acknowledgements
Volv would like to thank all the contributors from the WODC EU 2022 Workshop and subsequently the various 1:1 sessions, which have helped us realise this Whitepaper, as well as those that have directly contributed and reviewed it. The following is a non-exhaustive list:
Koen Degeling (Lumen Value & Access), Henk Dieteren (Suvoda LCC), Veronica Lopez Gousset, MPH (HTAi), Michele Lipucci, PhD and Christos Sotirelis, PhD (EURORDIS), Elin Haf Davies (Aparito), Dr. Fátima Núñez PhD (Hospital Sant Joan de Déu Barcelona), Robert Pleticha & Philipp von Gallwitz (Admedicum), Jan Willem Schmitz and Sophie Schmitz (Partners4Access).
[i] Parexel’s Deb Pasko shares her personal rare disease story in honour of Rare Disease Day, February 2023, https://www.parexel.com/news-events-resources/blog/parexels-deb-pasko-shares-her-personal-rare-disease-story-honor-rare-disease-day?utm_campaign=PostBeyond&utm_source=LinkedIn&utm_medium=366959&utm_term=Parexel%E2%80%99s+Deb+Pasko+shares+her+personal+rare+disease+story+in+honor+of+Rare+Disease+Day
[ii] Loulou Foundation announces First-Patient-In for CANDID observational study on CDKL5 Deficiency Disorder, October 2022, https://www.businesswire.com/news/home/20221031005413/en/Loulou-Foundation-announces-First-Patient-In-for-CANDID-observational-study-on-CDKL5-Deficiency-Disorder
[iii] Solving the unsolved rare diseases in Europe, Nature, June 2021. https://www.nature.com/articles/s41431-021-00924-8#:~:text=Around%2050%20percent%20of%20patients%20with%20a%20rare,%28WES%29%20is%20applied%20routinely%20as%20a%20diagnostic%20approach
[iv] The National Economic Burden of Rare Diseases Study, EveryLife Foundation and Lewin Group, February 2021. https://everylifefoundation.org/wp-content/uploads/2021/02/The_National_Economic_Burden_of_Rare_Disease_Study_Summary_Report_February_2021.pdf
[v] Rare Diseases, WHO, https://www.who.int/standards/classifications/frequently-asked-questions/rare-diseases
[vi] From internal Volv data, https://www.volv.global/other-products/inverse/ms-results
[vii] HMA-EMA Joint Big Data Taskforce Phase II report: ‘Evolving Data-Driven Regulation,’ HMA and EMA, 2019, https://www.ema.europa.eu/en/documents/other/hma-ema-joint-big-data-taskforce-phase-ii-report-evolving-data-driven-regulation_en.pdf