Contextualising Clinical Challenges: The History of Medical Knowledge
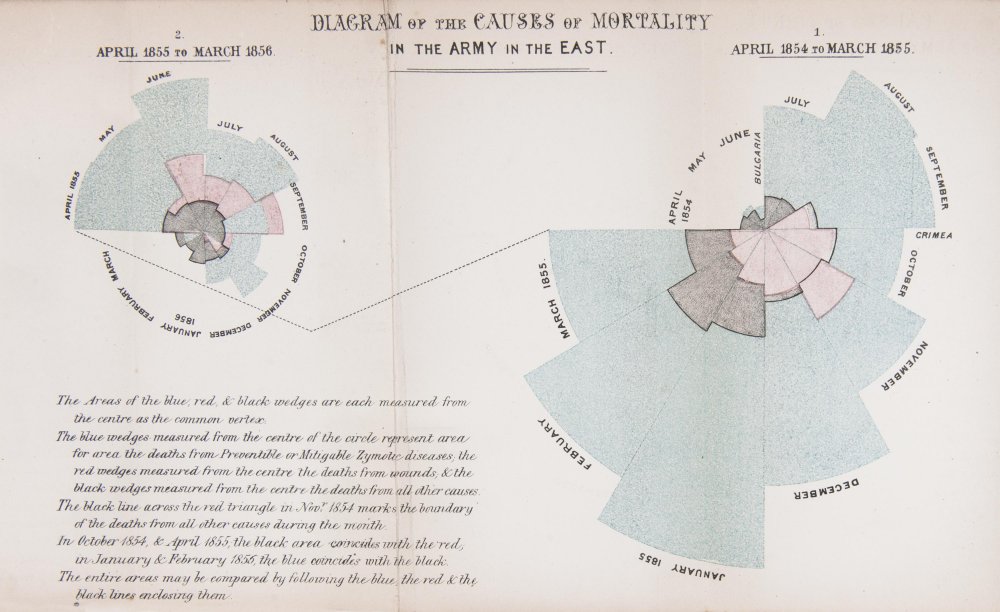
When Florence Nightingale returned from the Crimea to London in 1856, she set about publicising her statistical findings as well as her proposed medical reforms. But she was aware of the limited effect one person could have on practices within the armed forces and the nursing profession.
The first year (shown on the right of the diagram) was 1854–5, following her arrival in the region. The second (on the left) was 1855–6, after she had implemented a series of reforms to the hospital and nursing practices.
In her diagram, each wedge represented a month, and the area of the wedge showed the number of soldiers who had died that month. The blue area showed deaths from preventable diseases picked up in the terrible conditions at the Crimea. Red sections showed deaths from battlefield wounds. Black areas were deaths from other causes.
Readers could see two things. The first was that the reforms Nightingale implemented and campaigned for had made a huge positive difference to mortality. The second, and more shocking result, was that more soldiers died from preventable diseases during the war than from injuries.
Today, we are used to seeing statistics presented in graphical form. Infographics are common in newspapers, magazines and online. However, in 1850s Britain, the approach was revolutionary.
Credit: Thanks to the Science Museum, London for the inspiring image above and content.
The history of medicine
The practice of medicine has been around for millennia. From the Sumerian dynasty to China to Egypt to India to Ancient Greece, societies have shared traditions and knowledge about managing disease and healing patients. Even today, modern medicine adheres to some ancient traditions, in particular the Hippocratic Oath, written in Greece between the fifth and third centuries BC.
While we still apply some practices from early medicine, albeit under much safer and more hygienic conditions – Caesarean section for example – it wasn’t until after the Industrial Revolution and the scientific discoveries and inventions of the 19th Century that modern medicine really began.
Over the past 200 years, medical knowledge has accelerated with richer, more detailed information for healthcare practitioners to absorb and apply. From Louis Pasteur and the beginnings of medical microbiology to Florence Nightingale and how she completely changed hygiene practices in medicine. Nightingale’s influence on the way we capture data and assess it in modern medicine is as significant as her role in highlighting unsanitary healthcare conditions. Her mathematical background and commitment to gathering statistics might be described as the foundation of evidence-based medicine.
The 20th Century saw a rapid advance in medical discovery and knowledge. The discovery of the double helix structure of DNA by James Watson and Francis Crick was the foundation for modern molecular biology and led to the techniques on which the biotechnology industry is founded, including recombinant DNA research, gene sequencing and monoclonal antibodies. More recently, the medical and research worlds were transformed by the Human Genome Project, the world’s largest collaborative project, which set out to determine the base pairs that make up human DNA and improve tools for data analysis.
Medical breakthroughs in the past year alone have been extraordinary – from oncology advancements to new treatments that could profoundly change the prognosis for conditions such as atrial fibrillation, to gene editing that could enable specialists to cut any kind of DNA molecule at a designated location and potentially remove genetic errors. The mRNA vaccine technology, which has been studied for decades, can be developed in a laboratory using readily available materials, meaning the process can be standardised and scaled up far quicker, making future vaccine development faster than traditional methods of making vaccines. It has been instrumental in changing the course of the Covid-19 pandemic.
Keeping up with change
Medical advances, however, require knowledge of the condition they address, and therein lie many challenges. Knowing how to classify diseases, find them in patients, pinpoint different sub-categories, and identify heterogeneity of symptoms has long proven a problem.
Just knowing what is out there is a challenge. For example, how many diseases are there in the world? The answer is it keeps changing based on current knowledge. A recent data scrape of Orphanet found 9,332 unique rare diseases and 21,582 synonyms. According to a Washington Post fact checker article from 2016, there is no real consensus on how many diseases there are, although “a credible case can be made that there are at least 10,000 diseases in the world, though likely more.”
Growing knowledge about disease means the number of diseases catalogued keeps changing. A good example from mainstream diseases is high cholesterol. Once thought of as one condition, knowledge of the role cholesterol plays in health changed with the discovery of “good” and “bad” cholesterol. More recently, it has been found that there are five types of cholesterol low-density lipoprotein (LDL), high-density lipoprotein (HDL), triglycerides, very low-density lipoprotein (VLDL) and Lp(a) cholesterol, Lp(a) is a genetic variation of LDL. However, now thinking has moved from cholesterol as a major factor in cardiovascular disease (CVD), to insulin resistance as being a more significant factor. This knowledge has only emerged during the last few years, and so some people – patients and medical professionals – have been left behind, confused, and lacking in trust in what to believe. In rare diseases, those sub-categories are even more prevalent. As an example, Duchene Muscular dystrophy for example, where there are now 9 sub-types defined and categorised.
One disease that we have experience working on and that is notable for its many sub-categories is lipodystrophy. On Orphanet there are 31 unique forms of related diseases categorised with a unique disease identifier, which have 38 synonyms, although generally lipodystrophy is classed into two or three subtypes. It is a rare lipid storage disorder that is characterized by a loss of adipose tissue, lipodystrophy can be inherited or acquired as a result of medication or autoimmune illness. As a genetic illness it is extremely rare, with as few as 300 to 500 congenital generalized lipodystrophy (CGL) cases and 1,000 familial partial lipodystrophy (FPLD) cases. However, as is the case with many rare diseases, there are thought to be three times as many people suffering with the condition. Knowledge about the disease and its different forms has grown since the 1990s when understanding of adipose tissue biology started to expand.
There are nine major forms of muscular dystrophy: |
Many more Lipodystrophy diseases listed on Orphanet |
Lipodystrophy diseases listed continued…(click on links below to find out about each disease) |
Generalized lipodystrophy-progeroid features-severe intellectual disability syndrome |
||
Table1: Disease classification and synonyms continue to increase in complexity
This one example demonstrates the vast, complex, and changing nature of medical knowledge. Indeed, the depth of knowledge that healthcare professionals and life sciences professionals would need to have, store, and use to understand disease and sub-categories of disease, to develop therapeutics for those diseases, and to accurately diagnose and treat patients far exceeds what the human brain can retain.
Addressing the burden of diagnosis
To help healthcare professionals and the life sciences industry make sense of all this knowledge, Volv has used statistical and data science techniques to devise a path for recognising and assimilating relevant disease knowledge. In this way, doctors and researchers can find information that is relevant without having to sift through reams of data that isn’t pertinent to the problem at hand.
Essentially Volv is solving unpredictable events, finding data that was completely unknown to the person or organisation looking for patients or disease-specific information. What makes the approach unique is the machine learning is done without the need to have medical specialists annotate patient examples according to their phenotype (e.g., risk level or disease status). Volv’s ‘lightly-supervised’ learning is therefore much less expensive and labour-intensive than standard techniques, and it is also typically more accurate as it is not vulnerable to clinician-bias. Accurate learning from light-supervision is enabled by novel methods for detecting weak signals in vast amounts of data.
Future-proofing knowledge
As understanding of the data and patterns within the data grows, it allows researchers and HCPs to take different actions, which interrupt the data and remove clues, which in turn requires machine learning algorithms to adapt and find new clues. For example, if we find clues about mismanagement or misdiagnosis of a rare disease and the intervention corrects the management of the disease, those clues now disappear so the algorithm needs to find new clues.
Medical knowledge never stops evolving, so the machine learning and artificial intelligence algorithms that are developed to search for, identify and classify different diseases and disease sub-categories must also continue to evolve. Like the history of medical knowledge, good algorithms remain a work in progress.
Like Florence Nightingale, it takes new eyes, new vision to see the future, clarity of purpose and a need to make a change for the better, it's time to be inspired!